The History of Artificial Intelligence
by Rockwell Anyoha
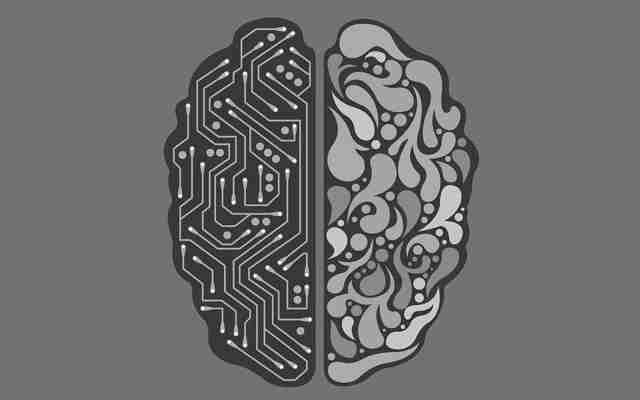
Can Machines Think?
In the first half of the 20th century, science fiction familiarized the world with the concept of artificially intelligent robots. It began with the “heartless” Tin man from the Wizard of Oz and continued with the humanoid robot that impersonated Maria in Metropolis. By the 1950s, we had a generation of scientists, mathematicians, and philosophers with the concept of artificial intelligence (or AI) culturally assimilated in their minds. One such person was Alan Turing, a young British polymath who explored the mathematical possibility of artificial intelligence. Turing suggested that humans use available information as well as reason in order to solve problems and make decisions, so why can’t machines do the same thing? This was the logical framework of his 1950 paper, Computing Machinery and Intelligence in which he discussed how to build intelligent machines and how to test their intelligence.
Making the Pursuit Possible
Unfortunately, talk is cheap. What stopped Turing from getting to work right then and there? First, computers needed to fundamentally change. Before 1949 computers lacked a key prerequisite for intelligence: they couldn’t store commands, only execute them. In other words, computers could be told what to do but couldn’t remember what they did. Second, computing was extremely expensive. In the early 1950s, the cost of leasing a computer ran up to $200,000 a month. Only prestigious universities and big technology companies could afford to dillydally in these uncharted waters. A proof of concept as well as advocacy from high profile people were needed to persuade funding sources that machine intelligence was worth pursuing.
The Conference that Started it All
Five years later, the proof of concept was initialized through Allen Newell, Cliff Shaw, and Herbert Simon’s, Logic Theorist. The Logic Theorist was a program designed to mimic the problem solving skills of a human and was funded by Research and Development (RAND) Corporation. It’s considered by many to be the first artificial intelligence program and was presented at the Dartmouth Summer Research Project on Artificial Intelligence (DSRPAI) hosted by John McCarthy and Marvin Minsky in 1956. In this historic conference, McCarthy, imagining a great collaborative effort, brought together top researchers from various fields for an open ended discussion on artificial intelligence, the term which he coined at the very event. Sadly, the conference fell short of McCarthy’s expectations; people came and went as they pleased, and there was failure to agree on standard methods for the field. Despite this, everyone whole-heartedly aligned with the sentiment that AI was achievable. The significance of this event cannot be undermined as it catalyzed the next twenty years of AI research.
Roller Coaster of Success and Setbacks
From 1957 to 1974, AI flourished. Computers could store more information and became faster, cheaper, and more accessible. Machine learning algorithms also improved and people got better at knowing which algorithm to apply to their problem. Early demonstrations such as Newell and Simon’s General Problem Solver and Joseph Weizenbaum’s ELIZA showed promise toward the goals of problem solving and the interpretation of spoken language respectively. These successes, as well as the advocacy of leading researchers (namely the attendees of the DSRPAI) convinced government agencies such as the Defense Advanced Research Projects Agency (DARPA) to fund AI research at several institutions. The government was particularly interested in a machine that could transcribe and translate spoken language as well as high throughput data processing. Optimism was high and expectations were even higher. In 1970 Marvin Minsky told Life Magazine, “from three to eight years we will have a machine with the general intelligence of an average human being.” However, while the basic proof of principle was there, there was still a long way to go before the end goals of natural language processing, abstract thinking, and self-recognition could be achieved.
Breaching the initial fog of AI revealed a mountain of obstacles. The biggest was the lack of computational power to do anything substantial: computers simply couldn’t store enough information or process it fast enough. In order to communicate, for example, one needs to know the meanings of many words and understand them in many combinations. Hans Moravec, a doctoral student of McCarthy at the time, stated that “computers were still millions of times too weak to exhibit intelligence.” As patience dwindled so did the funding, and research came to a slow roll for ten years.
In the 1980’s, AI was reignited by two sources: an expansion of the algorithmic toolkit, and a boost of funds. John Hopfield and David Rumelhart popularized “deep learning” techniques which allowed computers to learn using experience. On the other hand Edward Feigenbaum introduced expert systems which mimicked the decision making process of a human expert. The program would ask an expert in a field how to respond in a given situation, and once this was learned for virtually every situation, non-experts could receive advice from that program. Expert systems were widely used in industries. The Japanese government heavily funded expert systems and other AI related endeavors as part of their Fifth Generation Computer Project (FGCP). From 1982-1990, they invested $400 million dollars with the goals of revolutionizing computer processing, implementing logic programming, and improving artificial intelligence. Unfortunately, most of the ambitious goals were not met. However, it could be argued that the indirect effects of the FGCP inspired a talented young generation of engineers and scientists. Regardless, funding of the FGCP ceased, and AI fell out of the limelight.
Ironically, in the absence of government funding and public hype, AI thrived. During the 1990s and 2000s, many of the landmark goals of artificial intelligence had been achieved. In 1997, reigning world chess champion and grand master Gary Kasparov was defeated by IBM’s Deep Blue, a chess playing computer program. This highly publicized match was the first time a reigning world chess champion loss to a computer and served as a huge step towards an artificially intelligent decision making program. In the same year, speech recognition software, developed by Dragon Systems, was implemented on Windows. This was another great step forward but in the direction of the spoken language interpretation endeavor. It seemed that there wasn’t a problem machines couldn’t handle. Even human emotion was fair game as evidenced by Kismet, a robot developed by Cynthia Breazeal that could recognize and display emotions.
Time Heals all Wounds
We haven’t gotten any smarter about how we are coding artificial intelligence, so what changed? It turns out, the fundamental limit of computer storage that was holding us back 30 years ago was no longer a problem. Moore’s Law, which estimates that the memory and speed of computers doubles every year, had finally caught up and in many cases, surpassed our needs. This is precisely how Deep Blue was able to defeat Gary Kasparov in 1997, and how Google’s Alpha Go was able to defeat Chinese Go champion, Ke Jie, only a few months ago. It offers a bit of an explanation to the roller coaster of AI research; we saturate the capabilities of AI to the level of our current computational power (computer storage and processing speed), and then wait for Moore’s Law to catch up again.
Artificial Intelligence is Everywhere
We now live in the age of “big data,” an age in which we have the capacity to collect huge sums of information too cumbersome for a person to process. The application of artificial intelligence in this regard has already been quite fruitful in several industries such as technology, banking, marketing, and entertainment. We’ve seen that even if algorithms don’t improve much, big data and massive computing simply allow artificial intelligence to learn through brute force. There may be evidence that Moore’s law is slowing down a tad, but the increase in data certainly hasn’t lost any momentum. Breakthroughs in computer science, mathematics, or neuroscience all serve as potential outs through the ceiling of Moore’s Law.
The Future
So what is in store for the future? In the immediate future, AI language is looking like the next big thing. In fact, it’s already underway. I can’t remember the last time I called a company and directly spoke with a human. These days, machines are even calling me! One could imagine interacting with an expert system in a fluid conversation, or having a conversation in two different languages being translated in real time. We can also expect to see driverless cars on the road in the next twenty years (and that is conservative). In the long term, the goal is general intelligence, that is a machine that surpasses human cognitive abilities in all tasks. This is along the lines of the sentient robot we are used to seeing in movies. To me, it seems inconceivable that this would be accomplished in the next 50 years. Even if the capability is there, the ethical questions would serve as a strong barrier against fruition. When that time comes (but better even before the time comes), we will need to have a serious conversation about machine policy and ethics (ironically both fundamentally human subjects), but for now, we’ll allow AI to steadily improve and run amok in society.
Rockwell Anyoha is a graduate student in the department of molecular biology with a background in physics and genetics. His current project employs the use of machine learning to model animal behavior. In his free time, Rockwell enjoys playing soccer and debating mundane topics.
This article is part of a Special Edition on Artificial Intelligence.
For more information:
Brief Timeline of AI
Complete Historical Overview
Dartmouth Summer Research Project on Artificial Intelligence
Future of AI
Discussion on Future Ethical Challenges Facing AI
Detailed Review of Ethics of AI
What Is Artificial Intelligence, and How Does It Affect Your Daily Life?
The future is here. Find out how artificial intelligence affects everything from your job to your health care to what you're doing online right now.
Artificial intelligence, better known as AI, sounds like something out of a science-fiction movie. It brings to mind self-aware computers and human-like robots that walk among us. And while those things are part of the overarching artificial intelligence definition and may exist in the future, AI is already a big part of our everyday lives. So, what is artificial intelligence, exactly? It’s complicated, but every time you use Siri or Alexa, you’re using AI, and that’s just the beginning of its practical applications.
“The main benefit of AI is that it can bridge the gap between humans and technology,” says AI researcher Robb Wilson. “AI will allow everyone to communicate with computers the same ways they communicate with other humans: through speech and text. This can have the massive benefit of putting the problem-solving capabilities of powerful technology in everyone’s pocket.”
If you’re curious about AI, you’re not alone. In a recent Reader’s Digest survey, 23% of respondents said they were interested in learning more about it. It’s an important topic because the future of AI will shape everything from the internet to medical technology to our workplaces—for better and for worse. While AI will open up a whole new world with real robots helping in ways you probably never imagined, we’ll also have to contend with a changing job market, as well as unintended AI bias. We spoke to technology experts to break it all down. Here’s what you need to know.
What does artificial intelligence mean?
In a nutshell, artificial intelligence is simply a machine that can mimic a human’s learning, reasoning, perception, problem-solving and language usage. An AI computer is programmed to “think,” and this process hinges on programming that is called machine learning (ML) and deep learning (DL).
With ML and DL, a computer is able to take what it has learned and build upon it with little to no human intervention. But there are a few key differences between the two. In machine learning, a computer can adapt to new situations without human intervention, like when Siri remembers your music preference and uses it to suggest new music. Deep learning, on the other hand, is a subset of machine learning inspired by the structure of the human brain, says Lou Bachenheimer, PhD, CTO of the Americas with SS&C Blue Prism, a global leader in intelligent automation. As you may have guessed, this helps it to “think” more like a person.
Essentially, machine learning uses parameters based on descriptions of data, whereas deep learning also uses data that it already knows. In a real-world application, deep learning might help a digital worker easily decipher and understand handwriting by learning a variety of writing patterns and comparing it with data about how letters should look. AI will also play a big role in the metaverse in the future.
The history of AI
In 1935, Alan Turing envisioned machines with memory that could scan that memory for information. That idea eventually spawned the first digital computers, and in 1950, Turing developed a method to assess whether a computer is intelligent. The Turing Test involves asking a number of questions and then determining if the person responding is a human or a computer. If the computer fools enough people, it is considered thinking or intelligent.
It wasn’t until 1955, however, that scientist John McCarthy coined the term “AI” while writing up a proposal for a summer research conference. McCarthy later became the founding director of the Stanford Artificial Intelligence Laboratory, which was responsible for the creation of LISP, the second-oldest programming language and the one primarily used for AI.
Today, we have all kinds of “thinking” computers and robots. Have any passed the Turing Test? Yes. In fact, a chatbot recently fooled a panel of judges into thinking it was a 13-year-old boy named Eugene Goostman. Google AI has also passed the test. Does that mean these computers are sentient beings? No. Many say that the Turing Test is outdated and needs to be revised as a way to determine if a computer is actually thinking like a human. Currently, no computer actually thinks like a human.
How does AI work?
This essentially boils down to how AI learns, and it’s a lot like how a parent might teach a child. “When it was young and immature, AI was trained using lots of rules and patterns, which made systems like IBM’s Deep Blue really good at chess,” says Wilson about the program that was able to beat grand master Garry Kasparov in a chess match in 1997. “As AI has matured, it’s been trained more through trial and error. The AI makes mistakes, and like a parent, humans provide it with course correction and necessary context. As AI gets better at certain things, some of the rules established early on can be removed (much like a child earning more independence), creating further opportunities for growth.”
Of course, it doesn’t have a human brain’s neurons. Instead, a computer uses programming given to it by a human, or its algorithms process data to learn.
AI’s ability to get smarter over time makes it capable of producing solutions for previously unsolvable or challenging problems, according to Beena Ammanath, leader of Technology Trust Ethics at Global Deloitte and author of the business guide Trustworthy AI. For example, AI can learn to see connections in data sets that are way too complex for humans. This can lead to innovations like engineering better traffic flow in cities or predicting health problems in large demographics of people, and it can work with virtual reality to create digital models and other immersive experiences.
What are the four types of AI?
Yuichiro Chino/Getty Images
Artificial intelligence comprises four different types of AI. These types are then subdivided into two distinct groups called strong and weak AI.
Types of AI
The four types of AI are reactive machines, limited memory machines, theory of mind machines and self-aware AI. Each is progressively more complex and gets just a little closer to being like the human mind.
Reactive machines: This is the most basic AI. These machines don’t have memories to draw upon to help them “think.” They know how things should go and can even predict how something might happen, but they don’t learn from their mistakes or actions. For example, the chess computer Deep Blue could predict its opponent’s moves, but it couldn’t remember past matches to learn from them.
This is the most basic AI. These machines don’t have memories to draw upon to help them “think.” They know how things should go and can even predict how something might happen, but they don’t learn from their mistakes or actions. For example, the chess computer Deep Blue could predict its opponent’s moves, but it couldn’t remember past matches to learn from them. Limited memory machines: The next advancement of AI, limited memory machines can remember and adapt using new information. Social media AI uses this technology when it recalls previous posts you’ve liked and offers up similar content. The information isn’t gathered to be used long-term, though, like with the human mind. It serves a short-term purpose.
The next advancement of AI, limited memory machines can remember and adapt using new information. Social media AI uses this technology when it recalls previous posts you’ve liked and offers up similar content. The information isn’t gathered to be used long-term, though, like with the human mind. It serves a short-term purpose. Theory of mind machines: Science hasn’t yet reached this phase of AI. With theory of mind, the machine is able to recognize that humans and animals have thoughts, emotions and motives, as well as learn how to have empathy itself. With humans, this ability allowed us to build societies because we could work together as a group.
Science hasn’t yet reached this phase of AI. With theory of mind, the machine is able to recognize that humans and animals have thoughts, emotions and motives, as well as learn how to have empathy itself. With humans, this ability allowed us to build societies because we could work together as a group. Self-aware AI: The most advanced form of AI, this describes a computer that has formed a consciousness and has feelings. At this point, machines will be able to think and react like humans, like what we see in sci-fi movies.
Strong AI vs. weak AI
Strong and weak AI are separated by how “smart” the AI has become. With strong AI (also known as artificial general intelligence or AGI), a machine thinks like a human. Weak AI, or narrow AI, is the dumber version—and the one we currently have. Experts are split on when we will achieve strong AI. Many experts believe that it could happen within the next 50 years, though some say there’s a small chance that it could happen in the next decade.
With strong AI, a computer could learn, empathize and adapt while performing many tasks. It could be used to create robot doctors or many other professions that take both emotional intelligence and technical ability that grows and evolves as the robot learns through experiences. This is similar to personal health-care companion Baymax in the movie Big Hero 6 or the public servant robots in the movie I, Robot.
Weak AI enables the machine to do a task with the help of humans. Humans are needed to “teach” the AI and to set parameters and guidelines on how the AI should respond to perform its tasks. Siri, Alexa, Google Assistant, self-driving cars, chatbots and search engines are all considered weak AI.
Artificial intelligence examples
Now that you know the answer to the question “What is artificial intelligence?” you might be wondering where it is. The fact of the matter is that AI is everywhere in our world. Here are just a few common ways you interact with it on a daily basis without even realizing it.
Gaming
One of the most famous examples of early AI was the chess computer we noted earlier, Deep Blue. In 1997, the computer was able to think much like a human chess player and beat chess grand master Garry Kasparov. This artificial intelligence technology has since progressed to what we now see in Xboxes, PlayStations and computer games. When you’re playing against an opponent in a game, AI is running that character to anticipate your moves and react. If you’re a gamer, you’ll definitely be interested in the difference between AR and VR—and how AI relates to both.
Cars
Another example of artificial intelligence is collision correction in cars and self-driving vehicles. The AI anticipates what other drivers will do and reacts to avoid collisions using sensors and cameras as the computer’s eyes. While current self-driving cars still need humans at the ready in case of trouble, in the future you may be able to sleep while your vehicle gets you from point A to point B. Fully autonomous cars have already been created, but they are not currently available for purchase due to the need for further testing.
Health care
Currently, doctors are using artificial intelligence in health care to detect tumors at a better success rate than human radiologists, according to a paper published by the Royal College of Physicians in 2019. Robots are also being used to assist doctors in performing surgeries. For example, AI can warn a surgeon that they are about to puncture an artery accidentally, as well as perform minimally invasive surgery and subsequently prevent hand tremors by doctors.
Plus, robots come in handy when organizing clinical trials. AI can pick out possible candidates much more quickly than humans by scanning applications for the right ages, sex, symptoms and more. They can also input and organize data about the candidates, trial results and other information quickly.
Comparison shopping and customer service
Don’t want to pay more? AI can help. “The insurance company Lemonade is a good example,” says Wilson. “They’re relative newcomers to the space but have already disrupted the business model used by old-guard insurance giants. Users have easy access to policies and policy information through an intelligent bot, Maya, who continually receives rave reviews from customers.” Lemonade claims their customers save up to 80% on their insurance costs with a paperwork-free signup process that takes less than 90 seconds.
Similarly, China’s Ant Group has upended the global banking industry by using AI to handle their data and deal with customers. “As the 2020s were about to dawn, Ant surpassed the number of customers served by today’s largest U.S. banks by more than 10 times—a stat that’s even more impressive when you consider that this success came before their fifth year in business,” notes Wilson.
The impact of AI in the workplace
One survey from 2018 found that 60% of the companies surveyed were using AI-enhanced software in their businesses. A few short years later, AI is everywhere in the workplace. From search engines to virtual assistants, and from plagiarism detectors to smart credit and fraud detection, there’s probably not an industry that doesn’t use some form of AI technology.
Though it’s hard to predict just how AI will be used in the future of work, it is already making the workplace more enjoyable and efficient by taking over more mundane tasks like data processing and entry. In a 2022 study by SnapLogic, 61% of workers surveyed said that AI helps them create a better home/life balance, and 61% believed that AI made work processes more efficient.
Pros of AI
The Industrial Revolution created machines that amplified the power of our bodies to move and shape things. The Information Revolution created computers that could process enormous amounts of data and make calculations blindingly fast. AI is performing dynocognesis, which is the process of applying power to thinking, explains Peter Scott, author of Artificial Intelligence and You and founder of Next Wave Institute, an international educational organization that teaches how to understand and leverage AI.
By essentially being a heavy-lifting machine for thought, AI has the power to advance industries like health care, medicine, manufacturing, edge computing, financial services and engineering. “With the right set of tools and diverse AI, we can harness the power of the human-to-machine connection and build models that learn as we do, but even better,” says Ammanath. It can also enhance the performance of 3D printing, not to mention eliminate human error in the process.
According to Ammanath, some benefits of AI include:
Identifying patterns through the analysis of vast amounts of complex information.
Using natural language processing to engage with people in more human-like ways. For example, it will be harder to tell if a chatbot is a human or a computer.
Expanding human capabilities, which will help to create new development opportunities and products. In the same way machinery helps humans lift heavy objects, AI will help humans think big thoughts.
Allowing companies to remove more human bias and improve security measures to increase transparency.
Cons of AI
Of course, some problems have popped up as we venture into this new territory. For starters, as AI capabilities accelerate, regulators and monitors may struggle to keep up, potentially slowing advancements and setting back the industry. AI bias may also creep into important processes, such as training or coding, which can discriminate against a certain class, gender or race.
“Overall, the tool using AI and its ethical implications or risks are going to depend on how it is being used,” says Ammanath. “There is no single set of procedures that define trustworthy AI, but valuable systems should be put into practice at each institution to prevent the risks of AI development and utilization, as well as to actively encourage AI to adapt as the world and customer demands change.”
Another barrier to AI is the fear that future robots with AI will take away jobs. Of course, just like with any other automation advancement, new jobs have been created to improve and maintain automations. According to research by Zippia, AI could create 58 million artificial intelligence jobs and generate $15.7 trillion for the economy by 2030.
Some jobs will be lost, though. According to that same research, AI may make 375 million jobs obsolete over the next decade. We’re already seeing some jobs disappear. For example, toll booths that were once run by humans have been replaced with AI that can scan license plates and mail out toll bills to drivers. And travel sites run by AI that can find you the best flight or hotel for your needs have almost completely obliterated the need for travel agents.
The biggest problem lies in the fact that newer jobs created by AI will be more technical. Those unable to do more technical work due to lack of training or disabilities could be left with fewer job opportunities.
What the future of AI holds
As AI progresses, many scientists envision artificial intelligence technology that closely mimics the human mind, thanks to current research into how the human brain works. The focus will be on creating more innovative, useful AI that is affordable.
Ethical AI creation will also be an important part of future AI development. “People are concerned about ethical risks for their AI initiatives,” says Ammanath. “Companies are developing artificial intelligence boards to drive ethical behavior and innovation, and some are working with external parties to take the lead on instigating best practices.” This guidance will ensure that remedies for issues like AI bias will be put into place.
Will the world ever have self-aware AI? Experts are split on this one. Some say that with current innovations, we might one day see a machine that feels and has real empathy. Others say that consciousness is something only biological brains can achieve. For this level of AI, only time will tell.
Now that you know the ins and outs of artificial intelligence, learn about Web3 and how it will affect the future of the internet.
Sources:
What Is Artificial Intelligence (AI)? Definition, Types, Goals, Challenges, and Trends in 2022 |
Artificial intelligence (AI) is defined as the intelligence of a machine or computer that enables it to imitate or mimic human capabilities. This article explains the fundamentals of AI, its various types, goals, key challenges, and the top five AI trends in 2022.
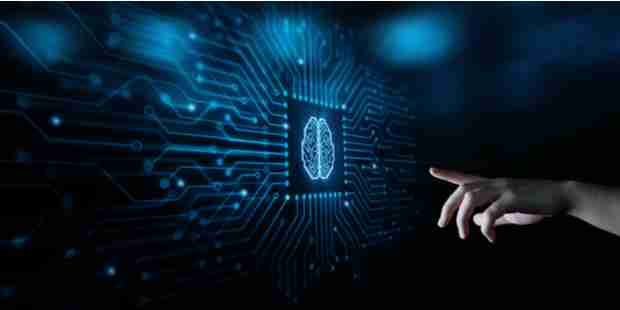
What Is Artificial Intelligence (AI)?
Artificial intelligence (AI) is the intelligence of a machine or computer that enables it to imitate or mimic human capabilities.
AI uses multiple technologies that equip machines to sense, comprehend, plan, act, and learn with human-like levels of intelligence. Fundamentally, AI systems perceive environments, recognize objects, contribute to decision making, solve complex problems, learn from past experiences, and imitate patterns. These abilities are combined to accomplish tasks like driving a car or recognizing faces to unlock device screens.
The AI landscape spreads across a constellation of technologies such as machine learning, natural language processing, computer vision, and others. Such cutting-edge technologies allow computer systems to understand human language, learn from examples, and make predictions.
Although each technology is evolving independently, when applied in combination with other technologies, data, analytics, and automation, it can revolutionize businesses and help them achieve their goals, be it optimizing supply chains or enhancing customer service.
How does AI work?
To begin with, an AI system accepts data input in the form of speech, text, image, etc. The system then processes data by applying various rules and algorithms, interpreting, predicting, and acting on the input data. Upon processing, the system provides an outcome, success or failure, on data input. The result is then assessed through analysis, discovery, and feedback. Lastly, the system uses its assessments to adjust input data, rules and algorithms, and target outcomes. This loop continues until the desired result is achieved.
How AI Works
See More: Top 10 AI Companies in 2022
Key components of AI
Intelligence has a broader context that reflects a deeper capability to comprehend the surroundings. However, for it to qualify as AI, all its components need to work in conjunction with each other. Let’s understand the key components of AI.
Key Components of AI
Machine learning: Machine learning is an AI application that automatically learns and improves from previous sets of experiences without the requirement for explicit programming. Deep learning: Deep learning is a subset of ML that learns by processing data with the help of artificial neural networks. Neural network: Neural networks are computer systems that are loosely modeled on neural connections in the human brain and enable deep learning. Cognitive computing: Cognitive computing aims to recreate the human thought process in a computer model. It seeks to imitate and improve the interaction between humans and machines by understanding human language and the meaning of images. Natural language processing (NLP): NLP is a tool that allows computers to comprehend, recognize, interpret, and produce human language and speech. Computer vision: Computer vision employs deep learning and pattern identification to interpret image content (graphs, tables, PDF pictures, and videos).
Types of AI
Artificial Intelligence can be broadly divided into two categories: AI based on capability and AI based on functionality. Let’s understand each type in detail.
Types of AI
Let’s first look at the types of AI based on capability.
1. Narrow AI
Narrow AI is a goal-oriented AI trained to perform a specific task. The machine intelligence that we witness all around us today is a form of narrow AI. Examples of narrow AI include Apple’s Siri and IBM’s Watson supercomputer.
Narrow AI is also referred to as weak AI as it operates within a limited and pre-defined set of parameters, constraints, and contexts. For example, use cases such as Netflix recommendations, purchase suggestions on ecommerce sites, autonomous cars, and speech & image recognition fall under the narrow AI category.
2. General AI
General AI is an AI version that performs any intellectual task with a human-like efficiency. The objective of general AI is to design a system capable of thinking for itself just like humans do. Currently, general AI is still under research, and efforts are being made to develop machines that have enhanced cognitive capabilities.
3. Super AI
Super AI is the AI version that surpasses human intelligence and can perform any task better than a human. Capabilities of a machine with super AI include thinking, reasoning, solving a puzzle, making judgments, learning, and communicating on its own. Today, super AI is a hypothetical concept but represents the future of AI.
Now, let’s understand the types of AI based on functionality.
4. Reactive machines
Reactive machines are basic AI types that do not store past experiences or memories for future actions. Such systems zero in on current scenarios and react to them based on the best possible action. Popular examples of reactive machines include IBM’s Deep Blue system and Google’s AlphaGo.
5. Limited memory machines
Limited memory machines can store and use past experiences or data for a short period of time. For example, a self-driving car can store the speeds of vehicles in its vicinity, their respective distances, speed limits, and other relevant information for it to navigate through the traffic.
6. Theory of mind
Theory of mind refers to the type of AI that can understand human emotions and beliefs and socially interact like humans. This AI type has not yet been developed but is in contention for the future.
7. Self-aware AI
Self-aware AI deals with super-intelligent machines with their consciousness, sentiments, emotions, and beliefs. Such systems are expected to be smarter than a human mind and may outperform us in assigned tasks. Self-aware AI is still a distant reality, but efforts are being made in this direction.
See More: What Is Super Artificial Intelligence (AI)? Definition, Threats, and Trends
Goals of Artificial Intelligence
AI is primarily achieved by reverse-engineering human capabilities and traits and applying them to machines. At its core, AI reads human behavior to develop intelligent machines. Simply put, the foundational goal of AI is to design a technology that enables computer systems to work intelligently yet independently. The essential goals of AI are explained below.
Goals of Artificial Intelligence
1. Develop problem-solving ability
AI research is focused on developing efficient problem-solving algorithms that can make logical deductions and simulate human reasoning while solving complex puzzles. AI systems offer methods to deal with uncertain situations or handle the incomplete information conundrum by employing probability theory, such as a stock market prediction system.
The problem-solving ability of AI makes our lives easier as complex tasks can be assigned to reliable AI systems that can aid in simplifying critical jobs.
2. Incorporate knowledge representation
AI research revolves around the idea of knowledge representation and knowledge engineering. It relates to the representation of ‘what is known’ to machines with the ontology for a set of objects, relations, and concepts.
The representation reveals real-world information that a computer uses to solve complex real-life problems, such as diagnosing a medical ailment or interacting with humans in natural language. Researchers can use the represented information to expand the AI knowledge base and fine-tune and optimize their AI models to meet the desired goals.
3. Facilitate planning
Intelligent agents provide a way to envision the future. AI-driven planning determines a procedural course of action for a system to achieve its goals and optimizes overall performance through predictive analytics, data analysis, forecasting, and optimization models.
With the help of AI, we can make future predictions and ascertain the consequences of our actions. Planning is relevant across robotics, autonomous systems, cognitive assistants, and cybersecurity.
4. Allow continuous learning
Learning is fundamental to AI solutions. Conceptually, learning implies the ability of computer algorithms to improve the knowledge of an AI program through observations and past experiences. Technically, AI programs process a collection of input-output pairs for a defined function and use the results to predict outcomes for new inputs.
AI primarily uses two learning models–supervised and unsupervised–where the main distinction lies in using labeled datasets. As AI systems learn independently, they require minimal or no human intervention. For example, ML defines an automated learning process.
5. Encourage social Intelligence
Affective computing, also called ’emotion AI,’ is the branch of AI that recognizes, interprets, and simulates human experiences, feelings, and emotions. With affective computing, computers can read facial expressions, body language, and voice tones to allow AI systems to interact and socialize at the human level. Thus, research efforts are inclined toward amplifying the social intelligence of machines.
6. Promote creativity
AI promotes creativity and artificial thinking that can help humans accomplish tasks better. AI can churn through vast volumes of data, consider options and alternatives, and develop creative paths or opportunities for us to progress.
It also offers a platform to augment and strengthen creativity, as AI can develop many novel ideas and concepts that can inspire and boost the overall creative process. For example, an AI system can provide multiple interior design options for a 3D-rendered apartment layout.
7. Achieve general intelligence
AI researchers aim to develop machines with general AI capabilities that combine all the cognitive skills of humans and perform tasks with better proficiency than us. This can boost overall productivity as tasks would be performed with greater efficiency and free humans from risky tasks such as defusing bombs.
8. Promote synergy between humans and AI
One of the critical goals of AI is to develop a synergy between AI and humans to enable them to work together and enhance each other’s capabilities rather than depend on just one system.
See More: What Is General Artificial Intelligence (AI)? Definition, Challenges, and Trends
Key Challenges of AI
AI is poised at a juncture where its role in every industry has become almost inevitable, be it healthcare, manufacturing, robotics, autonomous systems, aviation, and plenty others. However, just because AI holds enormous potential, it does not mean that one can ignore the numerous challenges that come along with it. The critical challenges for AI that businesses can recognize and work toward resolving to propel its growth are:
Key Challenges of AI
1. AI algorithm bias
AI systems operate on trained data, implying the quality of an AI system is as good as its data. As we explore the depths of AI, the inevitable bias brought in by the data becomes evident. Bias refers to racial, gender, communal, or ethnic bias. For example, today’s algorithms determine candidates suitable for a job interview or individuals eligible for a loan. If the algorithms making such vital decisions have developed biases over time, it could lead to dreadful, unfair, and unethical consequences.
Hence, it is vital to train AI systems on unbiased data. Companies such as Microsoft and Facebook have already announced the introduction of anti-bias tools that can automatically identify bias in AI algorithms and check unfair AI perspectives.
2. Black box problem
AI algorithms are like black boxes. We have very little understanding of the inner workings of an AI algorithm. For example, we can understand what the prediction is for a predicting system, but we lack the knowledge of how the system arrived at that prediction. This makes AI systems slightly unreliable.
Techniques are being developed to resolve the black box problem, such as ‘local interpretable model-agnostic explanations’ (LIME) models. LIME provides additional information for every eventual prediction, making the algorithm trustworthy since it makes the forecast interpretable.
3. Requirement of high computing power
AI takes up immense computing power to train its models. As deep learning algorithms become popular, arranging for an extra number of cores and GPUs is essential to ensure that such algorithms work efficiently. This is why AI systems have not been deployed in areas like astronomy, where AI could be used for asteroid tracking.
Moreover, complex algorithms require supercomputers to work at total capacity to manage challenging levels of computing. Today, only a few supercomputers are available globally but seem expensive at the outset. This limits the possibility of AI implementation at higher computing levels.
4. Complicated AI integration
Integrating AI with existing corporate infrastructure is more complicated than adding plugins to websites or amending excel sheets. It is critical to ensure that current programs are compatible with AI requirements and that AI integration does not impact current output negatively. Also, an AI interface must be put in place to ease out AI infrastructure management. That being said, seamless transitioning to AI is slightly challenging for the involved parties.
5. Lack of understanding of implementation strategies
Even though AI is on the verge of transforming every industry, the lack of a clear understanding of its implementation strategies is one of the major AI challenges. Businesses need to identify areas that can benefit from AI, set realistic objectives, and incorporate feedback loops into AI systems to ensure continuous process improvement.
Additionally, corporate managers should be well-versed with current AI technologies, trends, offered possibilities, and potential limitations. This will help organizations target specific areas that can benefit from AI implementation.
6. Legal concerns
Organizations need to be wary of the legal concerns of AI. An AI system collecting sensitive data, irrespective of whether it is harmless or not, might very well be violating a state or federal law. Although the data collected by AI may be legal, organizations should consider how such data aggregation can have a negative impact.
In January 2020, the U.S. government set forth draft rules for AI regulation. Some critical legal issues raised relate to civil liability. For example, if a driverless car injures someone in an accident, who is the culprit in such a scenario? Who takes responsibility? Such use cases raise the question of criminal culpability.
See More: How Is AI Changing the Finance, Healthcare, HR, and Marketing Industries
Top 5 AI Trends in 2022
As we dive deeper into the digital era, AI is emerging as a powerful change catalyst for several businesses. As the AI landscape continues to evolve, new developments in AI reveal more opportunities for businesses. Here are the top five AI trends and developments that will gain momentum in 2022.
Top 5 AI Trends in 2022
1. Computer vision set to grow
In the race for AI supremacy, organizations and businesses are set to embrace computer vision technology at an unprecedented scale in 2022. According to a September 2021 survey by Gartner, organizations investing in AI are expected to make the highest planned investments in computer vision projects in 2022.
Computer vision refers to AI that uses ML algorithms to replicate human-like vision. The models are trained to identify a pattern in images and classify the objects based on recognition. For example, computer vision can scan inventory in warehouses in the retail sector. Similarly, the technology finds application in several other industries such as healthcare, agriculture & farming, manufacturing, autonomous vehicles, and more.
2. Boost to the autonomous vehicle industry
As more and more car manufacturers continue to invest in autonomous vehicles, the market penetration of driverless cars is expected to rise considerably. According to Statista’s Dec 2021 projections, the global autonomous vehicle market is estimated to be valued at around $146.4 billion in 2022, a substantial rise from $105.7 billion in 2021.
Self-driving cars enabled with computer vision are already being tested by companies like Tesla, Uber, Google, Ford, GM, Aurora, and Cruise. This trend is only expected to scale in the next 12 months. In August 2021, Tesla unveiled the ‘Dojo’ chip specifically designed to process large volumes of images collected by computer vision systems embedded in its self-driving cars. Around the same time, Waymo, Google’s subsidiary, expanded its self-driving taxi services outside Arizona.
3. Chatbots and virtual assistants to get smarter
Another AI trend that is most talked about in 2022 is smarter chatbots and virtual assistants. This comes from the pandemic, as global industries are now comfortable giving their employees digital workplace experiences. Most chatbots and virtual assistants use deep learning and NLP technologies on the verge of automating routine tasks. Moreover, researchers and developers continue to add features and enhance these bots.
For example, Amelia, a global leader in conversational AI, performs complex conversation tasks with supplemental training provided by developers. Amelia claims to achieve 90% accuracy in identifying customer intent and a customer satisfaction rate of 91%, which is at par with human assistants. Tech companies such as Nuance, IBM, and Amazon Lex are making significant efforts to improve their virtual assistance through smarter bots.
4. Solutions for metaverse
AI agents and virtual assistants will play a key role as the tech world plunges into the concept of the metaverse. Metaverse defines a virtual environment that allows users to interact with digital tools and gives them an immersive experience. In October 2021, Mark Zukerberg rebranded Facebook as ‘Meta’ and announced plans to build a metaverse.
Virtual agents are expected to use AI to enable people to connect to the virtual environment. The famous humanoid AI robot Sophia is tokenized for metaverse appearance. Developers claim that tokenized Sophia, being AI, will interact with users from anywhere, at any time, and across devices and media platforms.
Although metaverse may not reveal itself in a full-fledged version in 2022, the blend of virtual and augmented technologies and AI will continue to stay as a backbone of the metaverse. Metaverse is therefore expected to be one of the major AI research trends in the next 12 months.
5. Improved language modeling
Another AI trend that will continue to feature in 2022 is improved language modeling. Language modeling is a technology that allows computers to understand language semantics, complete sentences via word prediction, and convert text into computer codes.
Generative Pre-trained Transformer 3 (GPT-3), by OpenAI, is a comprehensive language modeling tool available today. It uses 175 billion parameters to process and generate human-like language. Also, OpenAI, in August 2021, released a better version of its tool, Codex, which parses natural language and generates programming code in response. The company is also working on the next version of GPT-3 GPT-4), and it is expected that GPT-4 will be 500 times the size of GPT-3 in terms of the parameters that it may use to parse a language.
Apart from the trends listed above, other popular AI trends that could grab attention in 2022 include hyperautomation in modern businesses, the rise of artificial intelligence as a service (AIaaS), AI in cybersecurity, and increased sophistication in AIoT (merger of AI and the internet of things (IoT).
See More: AI Job Roles: How to Become a Data Scientist, AI Developer, or Machine Learning Engineer
Takeaway
As AI deepens its roots across every business aspect, enterprises are increasingly relying on it to make critical decisions. From leveraging AI-based innovation, enhancing customer experience, and maximizing profit for enterprises, AI has become a ubiquitous technology. This shift to AI has become possible as AI, ML, deep learning, and neural networks are accessible today, not just for big companies but also for small to medium enterprises.
Moreover, contrary to popular beliefs that AI will replace humans across job roles, the coming years may witness a collaborative association between humans and machines, which will sharpen cognitive skills and abilities and boost overall productivity.
Did this article help you understand AI in detail? Comment below or let us know on LinkedIn, Twitter, or Facebook. We’d love to hear from you!
MORE ON ARTIFICIAL INTELLIGENCE
Leave a Reply